Planificación e implementación de proyectos de sostenibilidad hídrica
En el oeste de Estados Unidos, diversas agencias y proveedores de agua están planificando e implementando proyectos de sostenibilidad de los recursos hídricos para responder al cambio climático y a la creciente demanda de suministro. En California, las Agencias de Sostenibilidad de las Aguas Subterráneas (GSA, por sus siglas en inglés) deben alcanzar y mantener la sostenibilidad de las aguas subterráneas para 2040, de conformidad con la Ley de Gestión Sostenible de las Aguas Subterráneas (SGMA, por sus siglas en inglés). Para afrontar este reto, las GSA están desarrollando proyectos como la reutilización del agua, la recarga gestionada de acuíferos, las transferencias de agua entre agencias y el almacenamiento y la recuperación de acuíferos (ASR, por sus siglas en inglés) para almacenar y utilizar eficazmente las aguas subterráneas.
La planificación eficaz de proyectos de sostenibilidad de aguas subterráneas que tengan en cuenta el cambio climático suele requerir el uso de un modelo de flujo de aguas subterráneas para simular los impactos de las configuraciones del proyecto con décadas de antelación. Estas simulaciones de modelos de aguas subterráneas pueden tardar horas o días en ejecutarse, y la optimización adecuada de los proyectos requiere numerosas simulaciones iterativas para identificar configuraciones mejoradas. Para acelerar la optimización de proyectos de sostenibilidad utilizando un modelo de aguas subterráneas, Montgomery & Associates (M&A) Desarrollamos un nuevo flujo de trabajo que utiliza algoritmos de aprendizaje automático (ML) para planificar, preprocesar, posprocesar y evaluar simulaciones de modelos físicos de forma autónoma. A este enfoque de flujo de trabajo lo denominamos Optimización Guiada por Aprendizaje Automático (MLGO).
Optimización guiada por aprendizaje automático
MLGO consiste en un proceso acoplado y automatizado donde los algoritmos de ML aprenden de las entradas y salidas de un modelo físico de flujo de agua subterránea para optimizar el diseño del proyecto y estimar combinaciones que cumplan con los objetivos de sostenibilidad. El flujo de trabajo de ML consiste en identificar automáticamente nuevas configuraciones y combinaciones de proyectos basándose en los resultados de modelos físicos previos, considerando los objetivos de optimización especificados por el usuario. Estos objetivos de optimización, definidos por el usuario, pueden reflejar los criterios de sostenibilidad deseados, los objetivos de viabilidad o las métricas de suministro. Este flujo de trabajo combina la eficiente capacidad de procesamiento de ML con la física real contenida en los modelos físicos de flujo de agua subterránea.
La siguiente ilustración describe cómo MLGO combina algoritmos de aprendizaje automático con un modelo físico de flujo de agua subterránea para optimizar los sistemas.
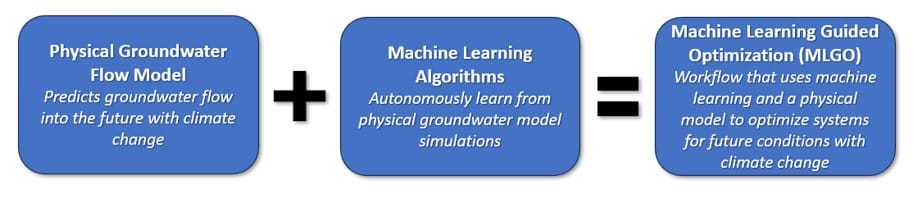
Estudio de optimización del agua regional del condado central de Santa Cruz
M&A utilizó MLGO para apoyar el Estudio Regional de Optimización del Agua del Condado Medio de Santa Cruz (Estudio). El objetivo del Estudio es respaldar la selección de proyectos de suministro de agua y acciones de gestión dentro de la Cuenca de Aguas Subterráneas del Condado Medio de Santa Cruz (Cuenca), con una sobreexplotación crítica, para operaciones a largo plazo y beneficios regionales compartidos, incluyendo la gestión sostenible de las aguas subterráneas y las necesidades regionales de suministro de agua. Los proyectos de suministro de agua y las acciones de gestión considerados incluyen ASR, la recarga de agua reciclada purificada (PWS) de Pure Water Soquel y las transferencias de agua entre agencias. Cada proyecto contiene numerosos parámetros de implementación, y la cantidad de posibles configuraciones es prácticamente ilimitada.
Para este trabajo, MLGO recibió capacitación sobre las entradas y salidas del modelo físico calibrado de flujo de agua subterránea existente y se le asignó la tarea de identificar configuraciones simuladas de proyectos que mejoren el suministro de agua regional, manteniendo al mismo tiempo la viabilidad y la sostenibilidad. Bajo la supervisión del modelador de agua subterránea y limitado por parámetros de implementación realistas definidos por el usuario, MLGO aprendió de cada simulación iterativa y progresó hacia la optimización del proyecto con el tiempo. Este proceso culminó con la identificación de cuatro alternativas robustas para la gestión del suministro de agua, que representan una gama de posibles inversiones en infraestructura y las mejoras asociadas al suministro de agua regional. El uso de MLGO contribuyó a la obtención de un producto final mejorado, ya que el proceso pudo avanzar de forma autónoma hacia la optimización y simular muchas más configuraciones de proyecto de las que habrían sido factibles con técnicas de optimización manual.
El siguiente esquema ilustra el flujo de trabajo general de MLGO, que progresa de forma autónoma hasta alcanzar la optimización.
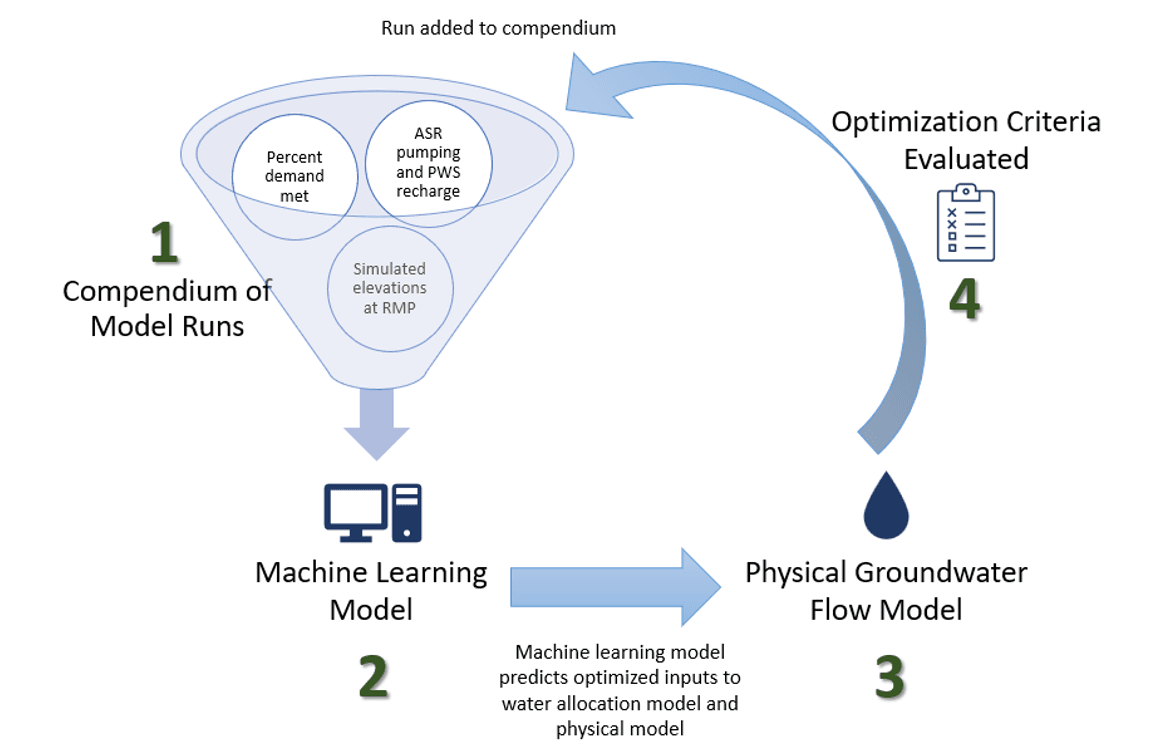
Otras aplicaciones
Además de ayudar a las GSA con la optimización del suministro de agua y la planificación de proyectos, los profesionales de fusiones y adquisiciones pueden utilizar MLGO para diversas aplicaciones de gestión de aguas subterráneas en entornos de recursos hídricos, minería y medio ambiente. Ofrecemos uno de los equipos de modelado de aguas subterráneas más grandes y experimentados del oeste de Estados Unidos, con más de 20 profesionales expertos en diversos códigos numéricos de flujo y transporte de aguas subterráneas. Nuestros modelos predictivos pueden incorporar incertidumbres futuras, como el cambio climático, o la estimación de la demanda futura de agua urbana y agrícola mediante escenarios o simulaciones probabilísticas. A menudo utilizamos modelos geológicos 3D para generar datos de entrada y presentar los resultados de forma accesible para las partes interesadas del proyecto. Nuestros expertos en SGMA son expertos en comunicar resultados de modelado complejos a las GSA y a las partes interesadas para apoyar la toma de decisiones a largo plazo para la gestión sostenible de las aguas subterráneas.
Sobre el Autor
Patrick Wickham, PG, es hidrogeólogo en la oficina de M&A en Pasadena y se especializa en modelado de aguas subterráneas y aplicaciones de aprendizaje automático. Patrick participará en el Simposio HydroML 2025, organizado por la Universidad de California-Irvine del 27 al 29 de mayo.